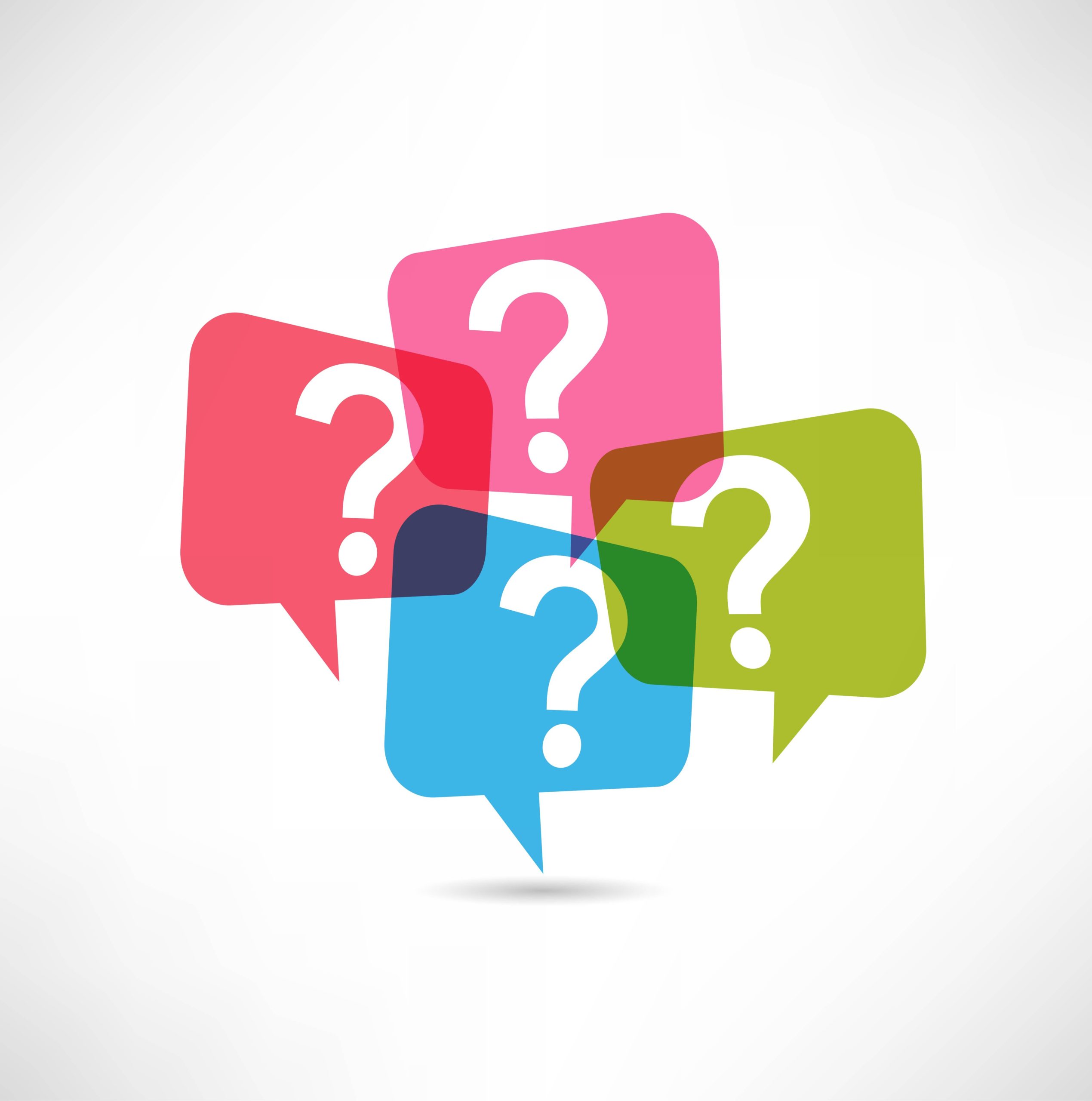
Forecasting: Let’s Start by Asking the Right Questions
Ten calls. They are all starting to sound the same. Here is the storyline: “We implemented SAP APO, and we now know its limitations. We
Ten calls. They are all starting to sound the same. Here is the storyline: “We implemented SAP APO, and we now know its limitations. We
Today hurricane Nate’s north winds are pushing against the bayous of Louisiana. In offices across the United States, demand planners are scratching their heads. The
“We have too many planners. And even if we can afford the costs, we don’t get good answers with our current forecasting processes,” stated the
Benchmarking is a measurement of the quality of an organization’s policies, products, programs or strategies against standard measurements. Business Dictionary.com Writing a Shaman blog post
This week I spoke at the Chicago CSCMP roundtable event. I love to hear the thoughts from different speakers. At the event, James Rice from MIT spoke
New shoes feel awkward. Blisters appear. Feet hurt. The shoes are worn for short periods. Often we shelve them to allow our feet to recover.
This week I interviewed Robert Byrne, Founder of Terra Technology, on the results of their fourth benchmarking study on forecasting excellence. For those that do not
It is Monday morning. As the sun rises, I find myself on the 6:00 AM train drinking coffee. I am giving thanks that I am able to do
On the first afternoon, it could be summed up as, “Oh father, we have sinned. Please forgive all of us sinners. “ This conference in
It happened yesterday. It happens at least once a day. When I travel, the most frequently asked question is “what do I see in the