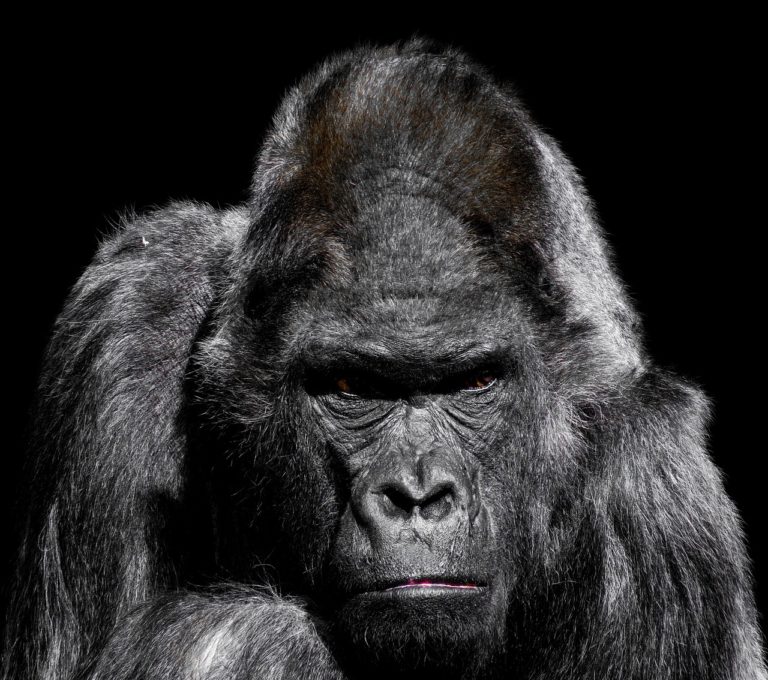
Getting Serious About Outside-in Processes
Within a supply chain organization there is turmoil. The enlightened leader knows that the efficient silos do not create the most effective supply chains; however,
Within a supply chain organization there is turmoil. The enlightened leader knows that the efficient silos do not create the most effective supply chains; however,
Over the past six years, I have studied the use of downstream data, and watched consumer products companies inch along, ever-so-slowly, with pilot projects. While I
It is 42 years old, and still not actionable. The first implementation was 1968 and we have talked about the integration of it to the supply
Last week, I was asked the question, “How do I take the steps to become more demand driven?” It prompted this response. Demand-driven value networks
It happens all the time. IT says to line of business leaders, “Tell me what you need for Business Intelligence (BI), and I will go
“We live in a world where supply chains, not companies, compete for market dominance. But companies often have diverging incentives and interests from their
It started as simple sales reporting. It is no longer simple. In 2005, there were five consumer product leaders actively using downstream data (retail inventory