I am writing this post from a hotel room in Chicago. My feet are swollen, and my luggage overflows with dirty laundry. I have not been home since the end of August. This week, I spoke at a University of Wisconsin event on responsiveness and ASCM on the future of supply chain planning. This speaking tour ends the first week of October.
Fall is conference season, and this year, with pent-up post-pandemic energy to return to events, I hit the trail to share insights on the value of outside-in supply chain planning along with recent research on the current state of supply chain management. While I am tired, shaking hands with others in-person at events feels good. While sometimes, I feel like the old lady in the room (many of my contacts are no longer active in the industry), the reception of the content by new supply chain leaders is positive, so I go on even though gray hair penetrates my hairline.
Here I want to share insights on my beliefs on the future of Supply Chain Planning. Let me start with what I think are “dead-end streets.” Let’s start with a definition.
“The end (as of a street) without an exit or a position, situation, or course of action that leads to nothing further.”
Miriam Webster
Dead-end Streets
At the beginning of the pandemic, no one imagined a period of thirty-two months of disruption. Supply chain leaders managed through disruption after disruption including increased weather impacts, energy outages, labor shortages, geopolitical shifts, and supply/logistics constraints. I forecast this disruption period as the new normal is not over. I believe that it will last for at least two-to-three years. Energy shortages and the lack of reliable power, the declining availability of water, commodity supply shortages, the migration of populations due to famine and war, and the lack of skilled labor top my list. In addition, COVID is not over. Vaccine effectiveness varies by region of the world so expect new variants.
Managing supply chains in the face of this level of uncertainty is new and unprecedented. At the beginning of the pandemic, the forecasted period of disruption of seven-to-eight months. (Seasoned supply chain professionals used the mental model from the recession of 2007 as a guidepost.) With the many M&A shifts and movement of supply chain talent due to death, retirement, and job changes, the world of supply chain leadership has more turnover and team instability than I have ever seen in my forty-year career.
Due to the need for urgency, I feel that we cannot waste our time, or our limited funds, on dead-end streets. Examples include projects like control towers, lights-out planning, real-time planning, DDMRP, forecast sharing, and sales forecasting. Instead, I think we should build outside-in planning processes to better use real-time and market data and build effective networks. Let’s examine each dead-end street:
- Control Tower. Most control tower implementations and RFPs lack a clear definition of what is being controlled. Instead, I find most are visibility projects without clarity on how to tie improved visibility to enterprise processes. So, if you are attempting to implement a control tower project, please don’t waste your money or resources. Here are some articles to read.
- Lights-out Planning. Lights Out Planning assumes that planning can be automated to not depend on the planner. While I believe that the explosion of the number of planners and the lack of a clear definition of the planner role is problematic, we are far from being able to implement a process that is not dependent on the trained planner. (The problem is the lack of trained planners.) Companies would be better served to measure plan adherence, plan quality, and process latency and train their planners while saving the time and energy of thinking about lights-out planning.
- Real-time Planning. Yes, the supply chain is awash with real-time data. Using real-time data is an opportunity for planning. Real-time channel and supply data should be used in the planning master data layer to update planning factors like lead times, conversion rates, yields, wait times, market prices, and availability. But this should not be translated into the misconception of running planning optimization more frequently. The reason is simple. More frequent running of the optimization engines injects noise into the complex, non-linear supply chain system. Since planning is both a means of optimizing the system and providing a system of record, the increased frequency of running planning engines will add to the confusion in an already confused state when the organization faces disruption after disruption. (For more on the building of the planning master data layer, reference the ASCM presentation from last week.)
- DDMRP. Using better math to build an inventory plan for raw materials is a good idea. The problem is that DDMRP assumes that the order is a good proxy for demand. As a result, DDMRP is a fad. (For additional reading check out this article.)
- Forecast Sharing. While running a supply chain based on the downstream customer’s forecast sounds good, most will find that most are not accurate enough by running an FVA analysis on the customer’s forecast. Test them yourself using the FVA technique. You will find that only a few customer forecasts will be helpful.
- Sales Forecasting. Again, asking sales to forecast sounds good, but the reality is that sales only know a piece of the market. While sales input is useful in sharing market drivers, sales forecasting tends to be biased based on sales and organizational incentives. In addition, sales forecasting processes add to process latency issues.
The Future
So, you might ask, what does the future of Supply Chain Planning will look like? No one knows, but here I share some insights based on testing.
I believe future applications are adaptive (reduced latency to translate market data into actionable insights) and distributed. In this transition, planners become orchestrators, and business leaders own planning outputs. In this transition we redefine work: the net result is fewer planners with greater job satisfaction. Planning taxonomies change to enable the trade-offs between source, make and deliver based on market signals and the design of supply chain flows to enable this shift.
- Adaptive. The engines within planning shift to embrace machine learning and artificial intelligence moving away from relational database architectures to embrace graph-based databases and ontological model representations. A planning master data layer informs these learning engines and continuously learns by mining insights from multiple inputs while mapping to multiple outputs. For example, in this modeling of LKQ data, machine learning improved the Forecast Value Added outputs while decreasing bias and bullwhip. The engine uses 11 inputs to map to five outputs ( Role-based demand visibility configured based on the needs of different team roles.) The engine learns based on the input and aligns the output while minimizing collinearity.
Table 1. Testing of an Outside-in Machine Learning Model based on Channel Data
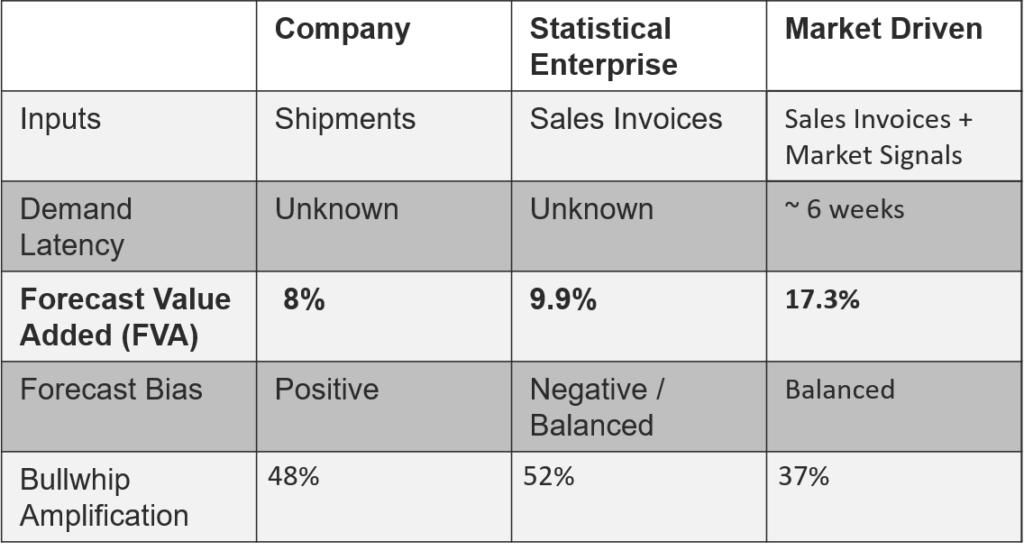
- Distributed and Democratized. Through the implementation of bi-directional orchestration with alignment to the balanced scorecard to the balanced scorecard. Bi-directional orchestration enables what-if optimization for commodity buy plans in procurement, S&OP execution, and transportation optimization.
Figure 2. Bi-Directional Orchestration
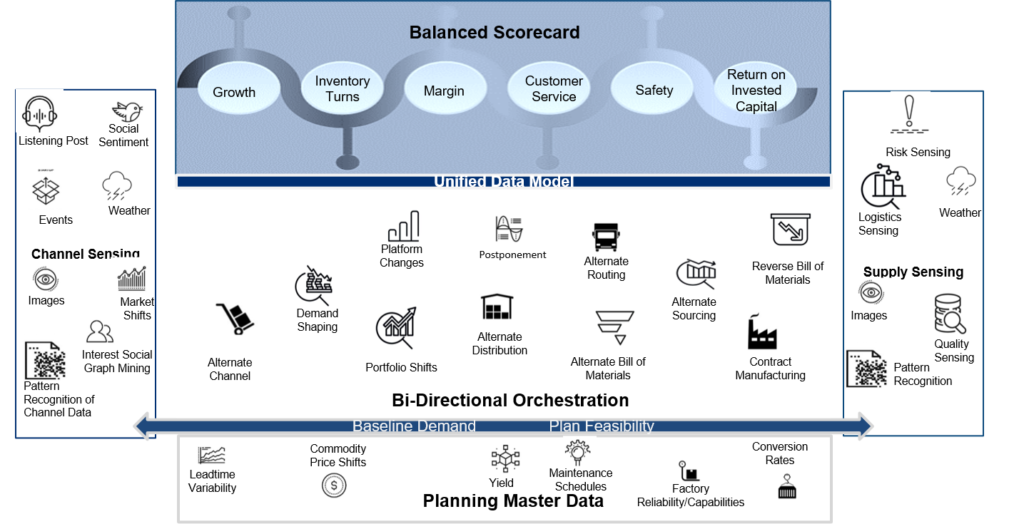
- Planning Taxonomy Redesign. These shifts introduce nine new capabilities into planning taxonomies redefining planning. This includes:
- Planning Master Data: Building a system to inform and align optimization engines based on market factors.
- Market-driven Demand: The use of market signals to decrease market and demand latency. Improving the time from market shifts to a decision.
- Demand Visibility: The generation of role-based outputs for all roles in the value network role-by role.
- Baseline Demand and Ongoing Analysis of Market Potential: Reflection of market potential with continuous analysis of demand shaping effectiveness while showing the impact of demand shifting.
- Unified Data Model Tied to a Balanced Scorecard: The movement from functional metrics to a balanced scorecard and the alignment of disparate planning engines through a unified data model.
- Continuous Design of Supply Chain Flows: Continous design of flows to enable decisions on nodes, form & function of inventory targets, procurement buy plans, transportation contracts, and manufacturing strategies. In the redesign, the design of flows proceeds S&OP processes and provides inputs to the multiple engines driving optimization.
- Procurement/Buyers Workbench. The design of a procurement workbench to consumer planned orders in the tactical horizon (outside of lead time) to implement buying strategies based on supply and demand variability and the bullwhip impact.
- Bi-directional Orchestration: The orchestration of market signals based on market signals and trade-offs to the balanced scorecard.
- Redesign of Transportation Planning to Deliver a Feasible Plan. Today’s transportation planning processes focus on cost mitigation and do not reflect the feasibility of the transportation plan while recognizing constraints.
Using the SCOR methodology, we built the level 1 view of this new approach to planning as shown in Figure 3. Over the next months, we will build out the level 2 and 3 definitions of these capabilities. This work is separate and distinct from the release of the SCOR 3 model today.
Figure 3. An Outside-in Planning Model
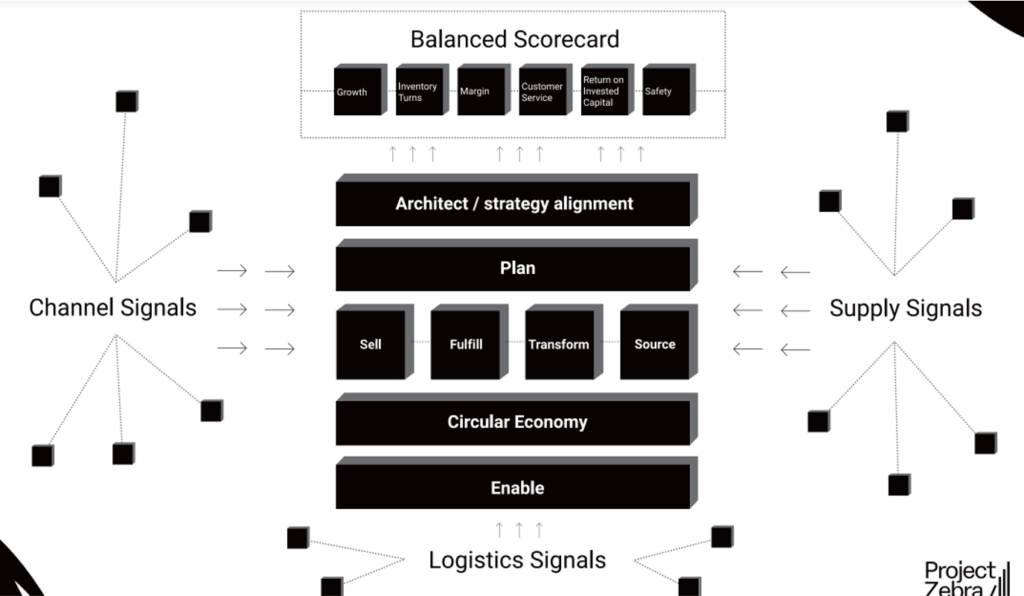
Conclusion:
It will take time to redefine planning to help in these times of heightened disruption, but it is clear that there is a need for a change, and testing is required. In the new model in Figure 3, note the alignment to a balanced scorecard (a shift from functional metrics) and the planning processes crossing both commercial and operations teams.
These concepts are new. Unlearning needs to proceed testing. But, to drive adoption, there needs to be a clear definition of value. This is the planned work. Follow the work at Zebra-org.