I dialed in, took a sip of coffee, and rocked back in my seat. I asked, “How can I help you?” (This is my standard modus operandi. When not traveling, I answer nine calls on average a day.) When I asked the question, an awkward silence followed. A strained request from a marketing director for a software company ensued, “You didn’t list us in your analytics report, and we want to know why.” I smiled. Why, you might ask? This type of inquiry is a compliment. How so? When people care enough about my writing, they ask this type of question if they want to know more. If they take the time to ask, then the writing and research matters.
The next comment made me laugh. The marketing director continued, “We are trying to understand. You see the world so much different than the Gartner analysts. They would have listed us.” At this point, I put the phone on mute and laughed. It was not a normal hee hee, but a deep belly laugh. I thought but did not verbalize on the phone, “Yes, of course. There is a reason why I am not a Gartner analyst.” But then I started thinking, had I been clear in the taxonomy of the recent supply chain analytics report? My answer was no.
In the 1990s, when I was part of the software team at Manugistics, supply chain analytics was a synonym for reporting. We were just starting to think of supply chain planning as decision support. Today, when you say the term analytics, the thought in many supply chain leaders’ minds is still ‘reporting’. It has also moved past the IBM marketing hype of Big Data Analytics. Thankfully, there is now more definition. Today, it is much, much more.
My goal for this blog post is to rectify my omission and give supply chain leaders a framework to better understand supply chain analytics. For the framework, I find five relevant dimensions:
First, Consider How to Drive Insights. Companies are data rich and insight poor. New forms of insights from predictive, to prescriptive, to cognitive analytics define new capabilities. Difference? While visualization helps business users to see the problem, there is no optimization. In predictive analytics, there is a clear objective function and a solve against a desired outcome. On prescriptive analytics, the solve yields exceptions and the insight engine gives recommendations on how to best manage them. Machine learning drives insights for prescriptive analytics. Cognitive sense, learn and then drive action. These engines are fed by sensing sensor data or through the use of unstructured text. We are moving up the stack from descriptive to predictive, to prescriptive to cognitive. The engines can be deployed in numerous types of analytics as shown in Figure 1.
Figure 1. Progressive Capabilities to Deliver Insights Through Analytics
Form and Function. As a supply chain planning gal, most of my posts focus on decision support. However, with new analytics, we can now improve workflows, collaboration, the use of sensors with streaming data, unstructured text mining, and the management of transactional data. Data can now move at the speed of business. While the traditional paradigm focused on batch and latent data, new forms of analytics redefine the Art of the Possible. Streaming data architectures are quite different from traditional analytic approaches.
Figure 2. Types of Analytics
A Third Element is the Type of Deployment. Analytics can be deployed in clouds, rivers or lakes. Traditional analytic approaches were more focused on reporting on applications like ERP, CRM, APS, WMS, and SRM. New approaches are not application specific. Instead, these analytic architectures sit between the traditional applications and workforce productivity.
A Fourth is the Database Structure. Moving to Schema on Read. New forms of analytics enable schema on read versus schema on write. The traditional use of use of relational database technology dictated schema on write forcing users to define hierarchies and relationships in deployment. The problem? Business changes. What is believed to be the right requirements in an early deployment might not be the answer. Schema on read enables a much more flexible approach for decision support and workflow. This approach makes many of the relational database structures questionable. While columnar store makes sense for transactional data that is used frequently, it is not a good choice for decision support for time phased data or streaming data architectures.
The Fifth Is Defining the System of Record. As analytic infrastructure evolves, less and less enterprise data will be written to ERP. More will be written to data lakes to enable schema on read approaches to enable new forms of process enablement. Transactional data, while important, will be combined with unstructured data more routinely in data lakes, clouds and streams. We must rewire our brains to not think so narrowly as the integration of transactional data into ERP as the end-to-end vision for supply chain management. Blockchain will become the System of Record for B2B. While there is much to test and evolve to enable multi-tier relationships, the technology is promising.
Recently, we published a report on analytics in our monthly newsletter. It is a popular subject. We had over 450 supply chain leaders sign up for our recent webinar.
In short, only one in four business users is satisfied with their current forms of analytics; but most companies struggle to drive an initiative to test-and-learn new forms of analytics using the three-part framework listed above. The most testing is in the area of visualization.
Figure 4. Current Levels of Investment
While blockchain and cognitive computing are believed to be the most disruptive within five years, less than 7% of companies are actively testing these technologies today. While immature, the two together offer great promise. We continue to push the envelope to help companies test these technologies through the share group for the network of networks. The next session is at the GS1 Headquarters in Chicago on April 4th-5th. Let me know if you are interested in joining the discussion and learning more.
Figure 5. Most Disruptive Analytics Strategies Within Five Years
So, in short, there are many facets to rethink analytics and drive business value through the Art of the Possible. It requires testing existing paradigms and redefining old processes. I hope this helps.
I look forward to getting your feedback. I am going to close now. Philadelphia streets are swarming with crowds celebrating the Superbowl win. I think I’ll go join the crowd.
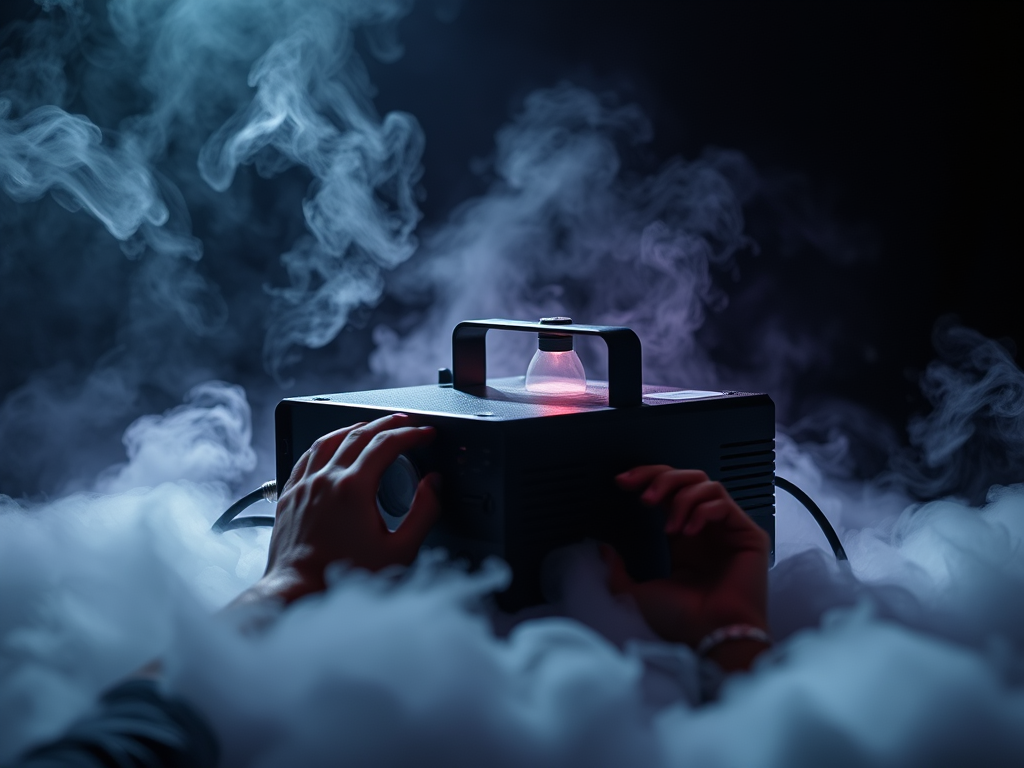
Turn Off the Fog Machine: Move Past Groupthink to Drive A Positive, Data-Driven Narrative
In this blog post, Lora Cecere, Founder of Supply Chain Insights, asks if our current focus on doom and gloom is a fog machine, and a barrier to driving improvement.