This blog post started a series of telephone calls. A large consumer products manufacturer with nine Enterprise Resource Planning (ERP) instances and several divisions wanted to discuss forecasting. The Center of Excellence at the company wanted to improve base-level capabilities but struggled to move forward due to the traditional views of the planning team, which they felt were self-serving. (The planning team was defending the status quo without questioning current practices and how to improve them.) The team was not calibrated on the role of forecasting and the basics around process excellence. The question was, “How can I redefine demand planning processes to use channel data?”
The next call was with a technology provider. The Company focused primarily on retail planning and wanted to extend its capabilities into a consumer products manufacturing solutions offering. They believed that retail data would help consumer products companies (which I agree with in concept) and that the retail forecast can be closely coupled–tightly integrated– to drive a better signal (which I disagree with). Their question was, “Why were consumer products companies not using retail data to drive demand processes?”
Upon hearing these questions, I sighed. I have been trying to answer these questions and help companies move forward for the past two decades but making little progress. The current state is a quagmire.
Quagmire: A situation that can easily trap you so that you become involved with problems from which it is difficult to escape. Definition
Merriam-Webster
In this blog, I try to answer both questions. As you will see, the answers are interrelated. But before I start, let me give you a disclaimer. This blog post may seem a bit basic for some. I find that in the industry, sometimes the basics get lost. Bear with me. I promise to answer the questions.
What Is a Forecast Anyway?
Let’s start with the basics. A forecast is a time-phased view, or projection, of future demand. In an implementation, the details matter. A forecast is not a forecast. They vary in time-frequency, planning horizon, granularity, and unit of measure. The taxonomies and goals are different.
In traditional advanced planning applications (APS) for a manufacturing company, the forecasting model’s role is to generate a time-series forecast in the tactical horizon (outside of lead time). (An easy way to think about time horizons is to align the thinking with supplying lead times. A model outside of lead time is tactical, whereas a model within lead time is operational, and a model within the order duration is an executional signal. Tactical processes are weekly or monthly, whereas operational processes are weekly or daily.)
In traditional planning taxonomies, the tactical forecast is modeled, and the operational signal is calculated using consumption logic. In 2004, with the evolution of demand sensing applications, the tactical and operational forecasts were both modeled using optimization, but the lack of synchronization of the two forecasts limited the technology adoption.
Using this logic, a grocery retailer’s forecast could project demand for 3-5 months (typical), while a consumer-packaged goods (CPG) company’s forecast could be 12-18 months, and a pharmaceutical company’s forecast could be 4-5 years. Starting to understand the issue with multi-tier demand planning?
Granularity also differs. A retailer’s forecast is usually in weeks, while a manufacturer’s forecast is typically a time-phased monthly forecast.
The planning hierarchies are also very different. A retailer is focused on shelf planning and assortment while a manufacturer is planning manufacturing supply and promotions.
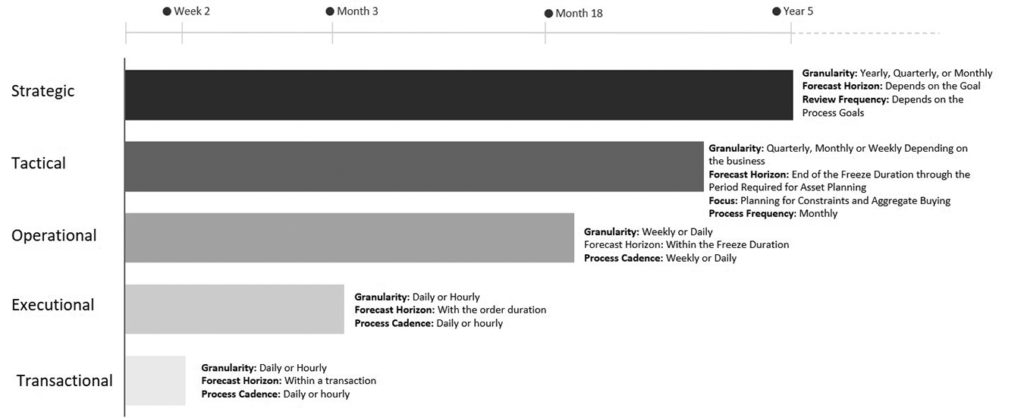
While the term demand sensing is used with abandonment in the industry (often over-used), it is seldom clearly defined. Many times, the term is confused with the use of market data as an input in a tactical demand forecasting model. Be careful. Don’t fall prey to discussions that are not grounded in the basic definitions. (Discussions devoid of definitions are the favorite ploy of the Supply Chain Conquistadors.)
Models Matter
In implementing planning, most of the focus in purchasing technology in the sales cycle is on optimization. Teams chose between technology approaches usually based on perceptions of optimization, software usability, cultural fit, and IT support of the integration layer. In this process, sadly, there is little definition of the model. However, based on my experience, model design is the most crucial discussion. (In this area, I find most companies having the wrong conversations. Companies should test multiple models using backcasting techniques after typing forecastability.)
Technology modeling capabilities are changing. As shown in Figure 2, traditional models used a single input to drive a single output. Companies used a single input–order or shipment data–to drive an output for the demand planner to be consumed into traditional supply chain planning. The demand signal is consumed into DRP to calculate safety stock and into tactical manufacturing planning to determine planned orders and identify constraints.
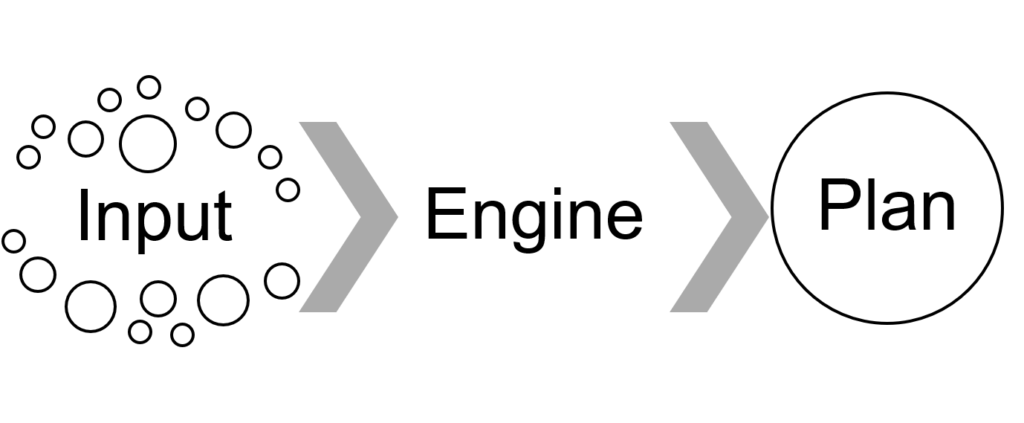
Could this change? The answer is yes. With the evolution of NoSQL–Graph and Cognitive Ontological learning–models can now manage multiple data sources as inputs while driving a forecast to multiple roles simultaneously in role-based views. This enables the modeling of channel data along with order and shipment data to drive a role-based view for finance, promotion planning, manufacturing planning (along with S&OP), transportation planning, and procurement. (These role-based views can be consumed into a demand visibility signal and used in the respective applications within transportation and procurement.) This evolution in technology makes the traditional views of price and promotion planning obsolete and could better synchronize supply. However, the approach is new and poorly understood, and only 7% of consumer products companies are innovators. Most are laggards comfortable continuing with a traditional approach. Unlearning is hard for teams, and most are rushing to implement it.
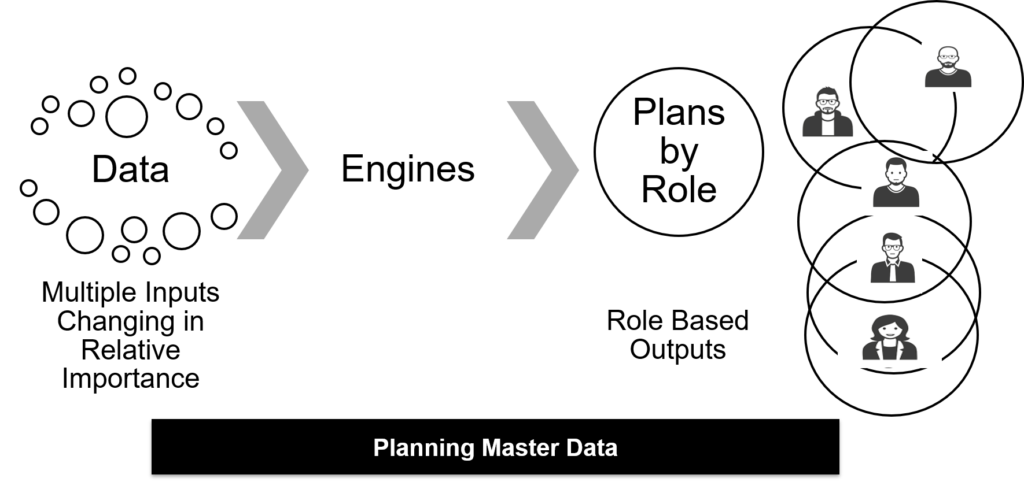
Model With the Goal In Mind
My favorite question to ask a group implementing demand planning is, “In an ideal world, what is the role of the forecast?” When I ask this question, the audience squirms in their seat. The reason? They don’t know the answer. By not asking this question and gaining clarity, companies limit their options. Let me explain.
The role of the forecast is different for a retailer and a manufacturer. While retailers use the forecast for inventory management and assortment planning, a manufacturer uses the forecast not only to manage inventory but also to align manufacturing capacities to manage constraints.
The most common deployment of forecasting technologies for manufacturing companies is a “ship-from model” mining patterns based on historical order or shipment data. (This type of model is shown as purple in Figure 4.) In a ship-from model, the locations are supply-centric by design. In a ship-to model, the locations in the model are channel-centric. (Companies continue to use history even though the past 36 months of disruption are a poor predictor of future demand.)
Less than 10% of companies forecast using a “ship-to” model (as shown in green). The evolution of ERP-centric planning, by definition, is a ship-from model. As a result, in most companies using traditional approaches to demand planning, there is nowhere to map channel data. Some companies with more mature modeling capabilities will move channel data as an indicator into a supply-centric model. However, only 12% of deployments have indicator capabilities, and only 31% of companies have what-if capabilities to view the impact of demand variation collaboratively.
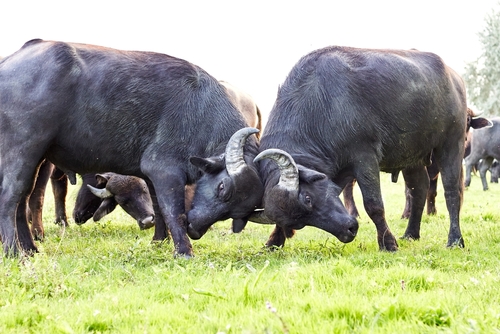
Demand is a tough subject. Organizations butt heads in the discussion of demand. The functions are not aligned and lack a shared understanding of demand management. Let me explain.
Channel data is used by sales account teams. The average consumer packaged goods manufacturer has 20-40 sales account teams that use channel data in various sales account planning technologies (often shadow IT). Each sales account team uses a different modeling technology. Their models are “ship to” models focused on sales account planning. They are often very retailer specific. The sales account team models run in isolation from supply chain planning. They are never synchronized, and in interviews, most companies lack an understanding of all of the applications used and models deployed.
Marketing teams typically use syndicated data with a latency of three-to-four weeks offset from channel consumption. The marketing processes are slower and forecasted at a category level (higher than the supply chain forecast of an item by location). Companies spend millions of dollars on shadow IT for sales and syndicated data for marketing. The trade and price models in sales and marketing do not align with supply chain processes which often means that companies will promote an item they cannot supply. Marketing plans typically carry a significantly positive bias. There is little discipline in marketing forecasting processes.
More advanced modeling using ontological representation and graph databases enables demand translation from a demand-side to a supply-side view. So, to generalize, manufacturers operate a supply-centric model while retailers operate a demand-centric model. While sales and marketing use channel data, the processes are isolated. There are significant issues in aligning sales and marketing processes with the supply chain work on demand. The best answer is often to start with a clean sheet of paper. The goal should never be a “one-number forecast.” (The issue is? A forecast, by definition, has many numbers, and in the future of demand planning, the models will generate synchronized views by role definition.)
In Figure 4, the lack of model alignment is a barrier across trading partners, making tight integration of forecasts fool’s play in traditional approaches. Companies need both demand (channel) and supply-side views by role.
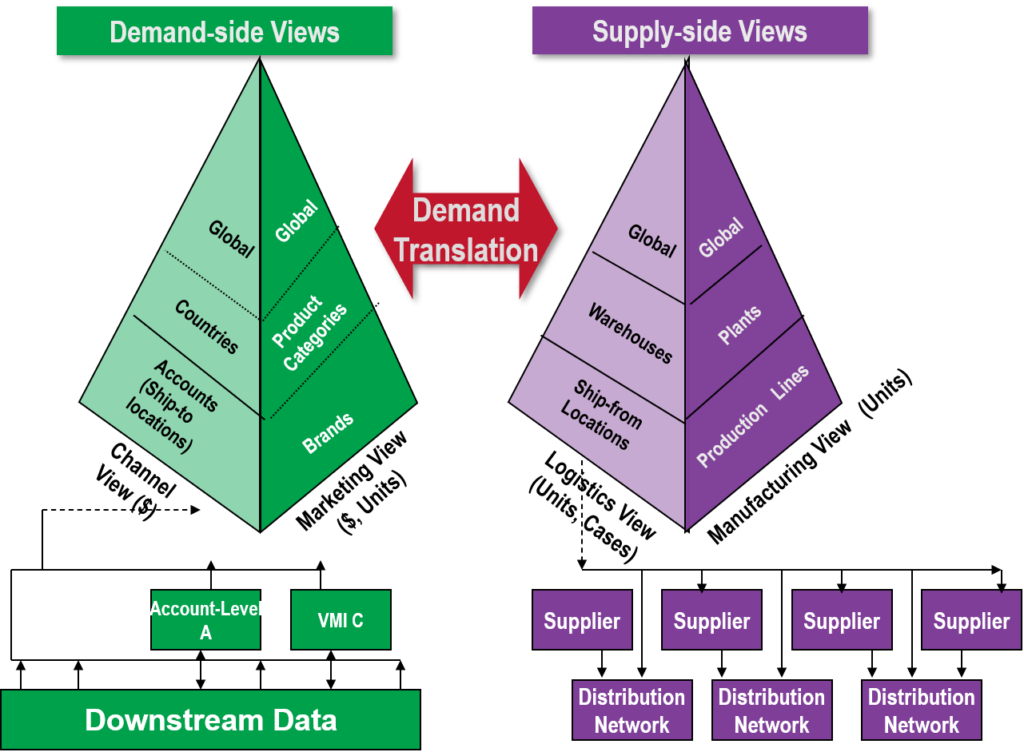
To best use channel and VMI data, a consumer goods manufacturer needs a “ship to” model and a level of demand translation for supply. Most technologists skip right over these modeling discussions and focus solely on optimization.
Unfortunately, in work on demand management, the industry has tried to make imperfect data precise. By definition, a forecast has error. Understanding the error and bias in forecasting is just as important, if not more important, than the forecast itself.
My Lessons Learned
Large organizations are very functional. Sales, marketing, and supply chain teams seldom discuss demand and why their modeling approaches cannot be synchronized. The world of technology is better at discussing engines or optimization. Companies seldom question in this new world, “What should be forecasted? What should be calculated?” Or, “How do I redefine models to decrease demand latency and improve responsiveness using channel data?” And “What could the role of the forecast be with new approaches in technology?”
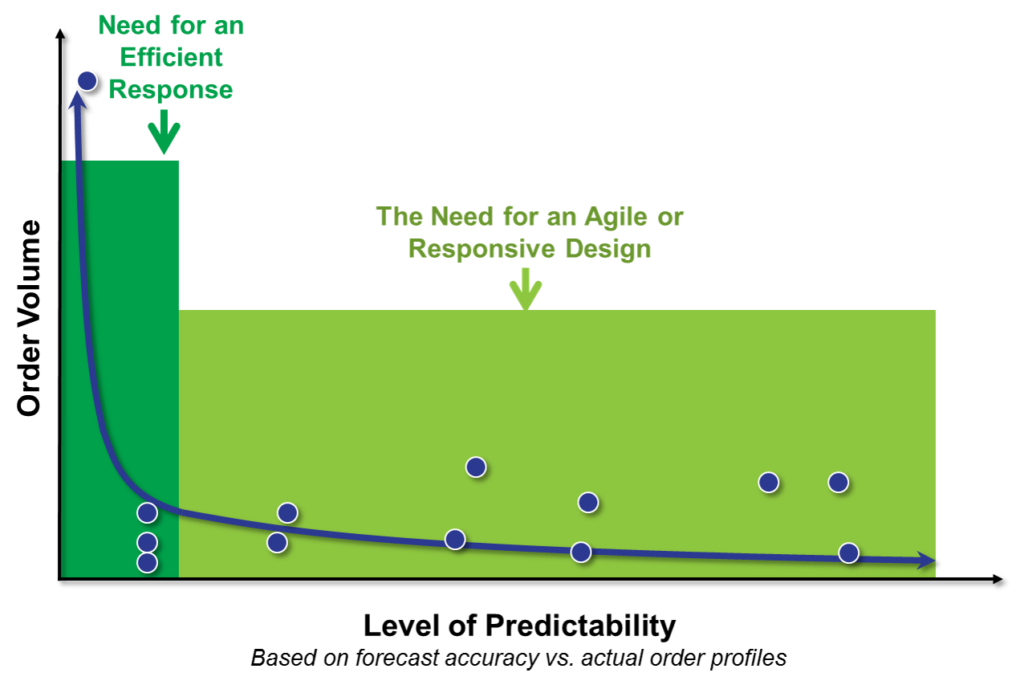
The evolution of the long tail of the supply chain over the past two decades is a reason to change. As shown in Figure 6, as products are added to the product portfolio, it is tougher to forecast an item and demand latency changes. (Demand latency is the time from shelf purchase to translate the sale through backroom replenishment to warehouse replenishment to a retail order to a manufacturer.) As a result, as the long tail increases, the order is less effective as a predictor of demand, making traditional approaches more problematic. The evolution of the long tail requires a rethinking of the model and an implementation of the system.
Misunderstandings
When I write, I love the comments. I thank the 331,000 readers on Linkedin who regularly share feedback on my work. The comments are an excellent barometer for me on the clarity of my writing and insight into the crowd’s wisdom. It also helps me to think more clearly. Here is my response to some recent comments on this topic:
- Flowcasting? Early in the testing of outside-in data, one planner commented that using channel data would reduce the need for forecasting and improve flowcasting. I scratched my head. Flowcasting, by definition, is flawed because not all products are designed to flow to the shelf based on regular replenishment. Think of the impact of demand shaping, seasonality, and new product launch. When products are promoted or advertised, the market potential of baseline demand shifts faster than the translation of demand based on order replenishment cycles. Also, the introduction of a new product introduces cannibalization within the category. In summary, the concepts of flowcasting are flawed because it never included the mapping of push-pull decoupling logic of demand shaping, seasonality, and new product launch.
- Tight Integration of CRM to Demand Planning? Tight integration is impossible due to the incongruities of the models. I have tried to do this for years.
- Throw away the APES. Most organizations fool themselves into thinking they have a good demand plan based on a simple calculation of Weighted MAPE. WAPE is a poor measure of success in demand planning. MAPE is only slightly better. Instead, measure Forecast Value Added (FVA). Sadly, I find that nine out of ten organizations that I work with using supply-centric models have a negative FVA. (This means that the expensive investments in demand planning teams and sophisticated optimization is degrading the forecast.)
- Redefine the Role of Syndicated Data. I wish that companies would cancel their contracts with syndicated data providers and invest in the use of channel data. Syndicated data is expensive, inaccurate, and increases the latency of process decisions (due to the demand latency of the signal). When I ask companies why they continue to spend millions of dollars on syndicated data, the answer is the analytics and the lack of understanding of the base issues. At a leadership level, there is a need to shift from a marketing-driven plan to a market-driven process to serve the shopper. Rethinking marketing processes within large CPG is like challenging religion, but it needs to be done.
- Bias. The management of bias becomes a big issue if the bias shifts suddenly. This happens with introducing a new set of incentives or management changes. Measure and understand bias by supply chain, taking action as it shifts.
- Just Drive Improvements in S&OP. S&OP is over-used and is not a panacea. No amount of effort in S&OP can ever overcome a bad demand management process.
- If I Use Channel Data, I No Longer Need to Forecast. Sigh. This is a symptom of not understanding how forecasts are used in manufacturing. Forecasts are used to manage inventory and align the organization to manage constraints. Constraints will never go away. If companies do not forecast, they cannot align.
- Don’t Assume That Functions Within A Companies Want to Share Data. In my research, I often find that sales and marketing teams do not want to share data with supply chain planning. The root issue is functional metrics. In many interviews, sales teams will share what they are doing if, and only if, I promise not to tell the larger organization. Shadow IT is alive and well.
- I Cannot Use Channel Data Because It Is Dirty or Incomplete. Second sigh. I believe it is time to embrace disparate data. New forms of analytics–machine learning, pattern recognition, and cognitive computing–helps us to get past this issue. The goal is to learn from the data before driving it as input into the model. In the planning master data layer, analyzing data completeness, integrity, and patterns help to overcome these issues.
Lessons For All:
As I wrap up this blog post, and finally answer the questions, there are five actions that I want to ask you to take:
- Forecast With the Goal in Mind. Ask yourself this question and answer it. Design the model to meet the goal.
- Understand the Impact of Demand Latency. Map the latency of the channel data to the order and understand how big of a barrier the dependency on order and shipment data for forecasting is for your organization by supply chain flow. (Most companies have five-to-six supply chain flows that are separate and distinct. A supply chain requiring a quick response should never be modeled using historical order data.)
- Carefully Design the Model. Work design should follow the model design. The design of the model is crucial. The role of planners and process definition should follow after the model’s design.
- Take Advantage of New Forms of Analytics. Invest in understanding the Art of Possible in-demand planning. Look beyond simple advances in optimization to understand what is possible using ontological learning and graph-based modeling.
- Have the Courage to Redefine Work and Question the Status Quo. Planning as we know it is labor-intensive and not effective in most organizations. Measure the current FVA and identify the issues. Use this discovery to help educate the team. Along with asking yourself the question of what is the role of the forecast, ask what should be the role of the planner? And is the current definition of work a barrier to the potential opportunity to redefine work using new forms of analytics?
A Succinct Answer to Both Questions
So, you might say, “OK. OK. Lora can you just answer the questions? Enough of the basics.” Let me try. (Sorry, I thought you needed some background.)
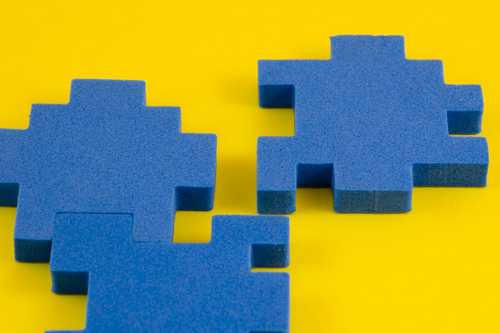
Why do consumer products companies not use retail data for supply chain planning? The answer is tradition. Companies lack alignment, and the pieces of software don’t neatly fit together. The rethinking of demand planning using new modeling techniques makes current Advanced Planning (APS), trade promotion, and sales planning techniques obsolete. Having the discussion requires the redefinition of work while challenging the status quo. Few companies have the leadership, understanding, and willingness to make this change.
Why should they make the change? The answer lies in empty shelves, higher inventory, and shipment reliability. As demand variability increases and the tail of the supply chain is elongated, demand latency (the time from shelf purchase to order signal to the manufacturer increases by weeks and sometimes months). As a result, companies are less responsive and agile. Currently, we see this issue in flu and cold vaccines. The shelves are bare. Companies in over-the-counter cold remedies primarily use traditional planning approaches using order and shipment data. Last year, we wore masks, and the incidence of flu was low. This year, the masks came off, and more of the population became sick. But the manufacturer was blind to the shift and the time for the response was too long. (The demand latency of shelf-takeaway to order in a normal season is 180 days. The supply chains are not designed to be responsive (short cycles and quick response). As a result, when the baseline consumer demand changes, the company is put on the back foot, losing sales.)
No question about it, the use of channel data improves demand accuracy by 40-60% while decreasing the bullwhip impact and demand latency. Consider the data from LKQ (an automotive aftermarket parts supplier) in Table 1. In the pilot testing over the past two years, we saw similar results with four manufacturers. Is this enough to change the market approach? Sadly no. The impetus remains to focus on supply-centric models using better optimization engines delivers only marginal improvements in results. The reason is tradition. It is hard to change an industry.
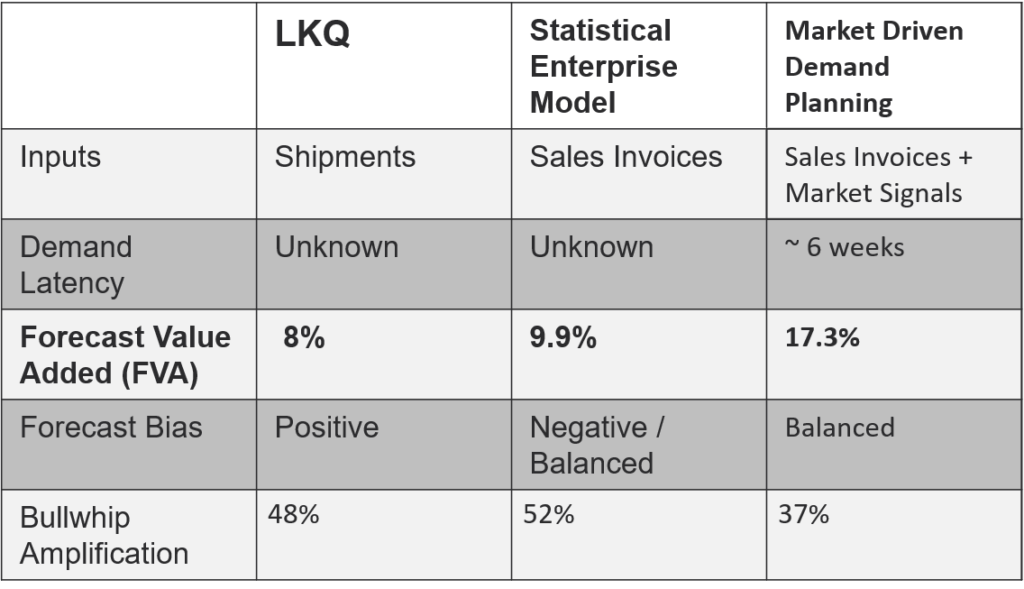
Why can consumer packaged goods companies not use retail forecasts? The first issue is relevance. The Forecast Value Added (FVA) of a retail forecast for a manufacturer is poor due to the differences in the planning horizon, granularity, taxonomy, and modeling. (Companies could use the VMI data if the demand models were built as Ship-to models, but they are not, so VMI data is not used by any large consumer manufacturer for supply planning. (Despite the alignment in granularity and model taxonomy.) VMI data only connects at order management.)
Whew! I know. A long answer to what seemed like a simple set of questions. I hope the explanation helps. Let me know your thoughts.
For me, the redefinition of demand planning is exciting and promising, but only if we can align on the current issues and unlearn the principles of existing processes. Today’s processes are fundamentally broken and a barrier for companies in a disruptive world.
Next Steps
Let me know if you are a manufacturer or retailer interested in testing new demand planning approaches using NoSQL and improved data models to improve demand planning. I am chairing a global collaborative share group to build a process definition to help companies effectively use channel data based on technological advances. My goal is to redefine work and improve supply chain planning processes. The group is only open to business leaders at manufacturing and retail companies. Seems like a shame that we are stuck in old ways, doesn’t it?
I look forward to getting your thoughts.